Flying Cars and Adaptive Drug Design: What are the Big Promises for Drug Discovery
April 18, 2013
By Allison Proffitt
April 18, 2013 | BOSTON—As the opening keynote at the 2013 Bio-IT World Conference & Expo, Andrew Hopkins started with the hard questions: Where are the flying cars?
The Chair of Medicinal Informatics at the University of Dundee, Hopkins is feeling a bit disenchanted. Ten years ago, when the Human Genome Project was completed, the field was full of the promise of new kinds of medicine and treatments—and cures!—for all kinds of diseases. Hopkins himself, formerly a chemist at Pfizer, coined the term “the druggable genome.” (see, Drug Discovery in Dundee)
But today there’s a pervading sense of disappointment. “Have we gotten the new types of medicine we were promised?” he asked.
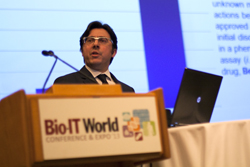
The economies of the orphan drug market are driving innovation in the space, thanks to changes in the efficacy vs population vs price equation. “People are realizing tradeoffs of risks relative to the market you’re going after,” Hopkins said.
But while the orphan drug market has been an area of growth and competition, the drug market as a whole has not blossomed as promised under the reign of genomics. New drug approvals ticked up in 2012, but generally Phase II and Phase III success rates have fallen. In a sobering statistic, the number of preclinical programs needed to produce a new drug grew from 12 five years ago to 30 today.
The problem, Hopkins says, is target validation. “We still have very little idea whether the biology we take into the clinic is likely to deliver a clinical differentiation.”
The druggable genome is increasing very slowly, said Hopkins (see, Cracking the Druggable Genome). There are some gene families with many targets—GPCRs, kinases—but most families are much smaller. As we keep discovering these smaller targets, the total number of drugs is increasing very slowly, he said, “even if each of those targets themselves are really exciting gene families to work with.”
Rather than expanding the druggable genome, Hopkins said researchers should focus on expanding the pharmacological playbook. The change, Hopkins proposes, is not really in the target landscape, but in our perception of how we modulate pharmacology.
Poly Perturbations
The shift will require a change in how we view a drug target. Rather than envisioning a single bull’s-eye, we should be looking to perturb the whole system, focusing on how many proteins work together. “Individual nodes by themselves are very hard to perturb,” Hopkins said, “only a few of them might be in the right position. But once you start multiple perturbations, you have a great chance of perturbing the system.”
For the drug hunter, Hopkins said, there are important lessons in systems biology and large-scale functional genomics. Study disease networks and identify related diseases. Look for redundancy—perturb both the chemical and genetic systems.
Claiming polypharmacology as a design strategy is not new. It was the basis of many central nervous system GPCR drugs designed in the ‘80s. And it’s not really a different type of problem, Hopkins argued. Drug design has always been multi-dimensional. Adding additional targets and navigating away from anti-targets may add more dimensions, but it doesn’t change the problem-type.
But successfully designing drugs this way does require tools. Hopkins and his team are taking an automated adaptive approach.
Creativity Automated
In 2004, a Japanese group of researchers studied how to automate creativity. They studied the way that humans think and approach creative challenges, and tried to mimic those processes computationally. They discovered that creativity as a cognitive process generates solutions to a task that are novel or unconventional and satisfy certain requirements. Define those requirements and you can tackle any problem computationally.
Hopkins chose to test the theory by attempting to evolve a new bioactivity from donepezil (Aricept), creating a potent dopamine D2 ligand.
Using interviews from medicinal chemists, the team looked at how researchers would design a compound. “We wanted to formalize the medicinal chemistry tactics,” Hopkins said. Once identified, the team ran the tactics against the whole ChEMBL database.
With a host of virtual compounds, the team ran an adaptive design algorithm to predict GPCR profiles, compared the results to experimental data, and narrowed the field. With each round of computational experiments, clarified design objectives refined the list of compounds.
The experiment was a success. After four rounds, the team had two compounds—21s and 27s. “We patented it just to prove a point,” Hopkins said, “that we could file intellectual property against compounds predicted by the algorithm.”
Hopkins was quick to point out that the computer is not listed on the patent. One assumes it is also not on payroll at Hopkins’s new company, ExScientia, founded to commercialize the technology.
Adaptive Continuum
The exercise proves that adaptive design works as a way of making use of the large datasets now available to us, Hopkins said. The algorithms can help us learn how to design drugs, and can even create intellectual property.
An even more interesting challenge though, is thinking about how applying adaptive techniques to drug discovery can build an entire adaptive pipeline—“a continuum of adaptive philosophies” that can be adapted from drug design to clinical trials.
Perhaps that would offer the “flying car” pharma has been missing.