Uncertainties in Assembly: Communicating and Managing the Truth About Our Data
August 26, 2013
By Allison Proffitt
August 26, 2013 | Published in late July in GigaScience, the Assemblathon 2 paper has been in the works since June 2011. Three genomes and about 17 GB of compressed data later (doubled if you include the contig files that were extracted from the scaffolds), the findings can be summarized in five words: “We’re doing it all wrong.”
At least according to C. Titus Brown. Brown is an assistant professor in the departments of Molecular Biology and Genetics; and Computer Science and Engineering at Michigan State University. Brown was an early reviewer of the paper, and took to his blog, Living in an Ivory Basement, soon after to hash out his thoughts.
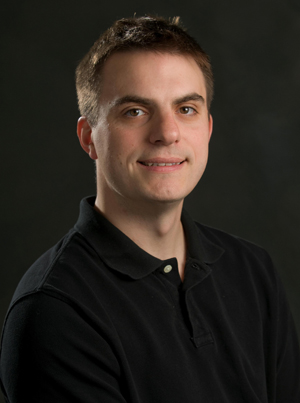
The Assemblathon had 43—16 fish assemblies for a Lake Malawi cichlid (dataset provided by Broad); 12 assemblies for a Red tailed boa constrictor (dataset provided by Illumina); and 15 assemblies for a Budgerigar or common pet parakeet (dataset provided by Erich Jarvis at Duke University and the BGI and Pacific Biosciences).
“There are many genome assembly programs out there, but it is not always clear as to which is the best,” the Assemblathon organizers explained in the published rationale for the project. “Part of the problem is that it is not easy to define what ‘best’ is and an assembler that might work well in one situation (e.g. assembling a high-repeat-content genome) might not fare as well in other situations,” they continued.
Brown said he wasn’t surprised that the assemblies were different. “I thought, ‘Well I would have expected that,’” Brown said. “But what I realized that it meant was that whenever somebody produced the assembly of something, they were failing to take into account that if you’d used a different technique, you might have gotten a subtly different answer. Until this paper came out, I hadn’t put that into words or really realized that.”
Of course we can’t judge which assemblies were “right” because we don’t know. “There may not be one answer,” Brown said. “The question isn’t, ‘Is the result correct?’ It’s, ‘Is the result useful?’”
For example, Brown was most struck by the analysis of core gene repertoires, “genes that every eukaryotic genome should have.” Each assembly showed about 95% of the catalog, a measure of how complete the assembly was.
“But the thing that struck me was that the 5% that was missing was different between the different genomes,” Brown said. “I would not have expected that. You could put them all together and get a 100% catalog, but they were each missing a slightly different subset of the core genes. I couldn’t really think of a really good, straightforward computational reason why that would happen.”
This presents a twofold problem, Brown believes. On the one hand, it’s not surprising to a bioinformatician that assemblies are different. Different assemblies address different goals—and that’s fine as long as you know that’s the case.
Yet as Brown points out in his blog, “we have been told, through a succession of papers in high profile journals and with all of the various genome browsers, that here is THE genome of mouse, here is THE assembly of zebrafish. As a result, the unwary biologist (which is many of them) will unwittingly trust the assembly we have.”
In Brown’s own experience working on the sea urchin genome, 8% of the raw reads never made it into the published assembly. “We said, ‘Here’s our final assembly. This is what we analyzed. It’s as good as it’s going to get for this paper. We’re done.’”
That’s commonly the case, he says, but it’s not commonly discussed. “It doesn’t make it into the paper; it doesn’t make it into the reader’s head. It doesn’t make it into the discussions between the old guard who largely decide a lot of the funding and initiative efforts,” Brown says.
Leaving out that data has real repercussions. “It short changes anyone who is actually trying to improve the genome,” by presenting an inaccurate view of what is completed.
“Biology computation is enough of a black box for enough biologists that they simply don’t realize how uncertain we really are,” Brown said.
He recounted a conversation over lunch with a colleague trying to choose the “right” option from three slightly different test results. “Why aren’t you content with just having three results?” Brown asked. “Because then we won’t know which result is the correct one,” was the response.
“Why,” Brown countered, “when you only had one result, did you think that was the correct one?”
It’s a paradigm that computer scientists are comfortable with, he said, but biologists are very wary of.
Once the uncertainty inherent in genome assembly is fully open, what can be done to address it? How does one work with 12 different boa constrictor assemblies from the same dataset?
“There are no good ways to combine those assemblies and no good ways to compare those assemblies. We’re really facing a lack of tools,” Brown said. “It’s much more exciting to write your own nifty tool that does something subtly different from what everyone else has done, than to actually do a good job of comparing and evaluating tools.”
Brown joked that any conversation with a scientist would inevitably return to funding, and proved his point by calling for funding to develop the tools needed to compare and combine the results we have. “It all goes hand in hand, right? We can’t get the funding to develop the tools until we have the cultural outlook that says building the tools is useful.”
It’s not just tools that are necessary, but the skills to tackle these problems as well.
“The goal of assembly projects is not just to generate the assemblies but to generate the population of researchers that can make use of them. Those skills are also what you learn when you have people involved in these software development projects.”
Projects like Assemblathon will drive the maturity of the field, Brown believes. “That’s going to be a lasting legacy of this paper. Who cares what assembly we were using in 2013, but we started to have a much more mature conversation about how we should compare genomes.”